Explicit Runge-Kutta methods exercise solutions#
Solutions to the exercises on explicit Runge-Kutta methods.
Solution to Exercise 2.1
Solution to Exercise 2.2
Solution to Exercise 2.3
The order conditions are:
Solving gives \(b_2 = \frac{2}{3}\), \(c_2=\frac{3}{4}\) and \(a_{21} = \frac{3}{4}\) so the method is
Alternatively as a Butcher tableau
from sympy import *
# Declare symbolic variables
a21, b1, b2, c2 = symbols('a21, b1, b2, c2')
b1 = Rational(1,3)
# Define order conditions
eq1 = b1 + b2 - 1
eq2 = b2 * c2 - Rational(1,2)
eq3 = a21 * b2 - Rational(1,2)
# Solve order conditions
solve((eq1, eq2, eq3))
% Declare symbolic variables
syms a21 b1 b2 c2
b1 = 1 / 3;
% Define order conditions
eq1 = b1 + b2 == 1;
eq2 = b2 * c2 == 1 / 2;
eq3 = a21 == c2;
% Solve order conditions
solve(eq1, eq2, eq3)
Solution to Exercise 2.4
Adding the labels to the tree
Solution to Exercise 2.5
The set of rooted trees up to and including order 3 are
Tree |
\(\Phi\) |
\(\gamma\) |
---|---|---|
\(\displaystyle\sum_i b_i\) |
1 |
|
\(\displaystyle\sum_i b_ic_i\) |
2 |
|
\(\displaystyle\sum_i b_ic_i^2\) |
3 |
|
\(\displaystyle\sum_{i,j} b_i a_{ij} c_j\) |
6 |
Using \(\Phi = \dfrac{1}{\gamma}\) we have
Since for an ERK method \(c_1 = 0\) and \(a_{ij} = 0\) where \(i \leq j\) then the terms in red are zero so the order conditions are (including the row sum conditions)
Solution to Exercise 2.6
Use Python or MATLAB to solve the order conditions
import sympy as sp
# Declare symbolic variables
a21, a31, a32, a41, a42, a43, b1, b2, b3, b4 = sp.symbols("a21, a31, a32, a41, a42, a43, b1, b2, b3, b4")
# Define c values
c2, c3, c4 = sp.Rational(1,4), sp.Rational(1,2), 1
# Define order conditions
eq1 = b1 + b2 + b3 + b4 - 1
eq2 = b2 * c2 + b3 * c3 + b4 * c4 - sp.Rational(1,2)
eq3 = b2 * c2 ** 2 + b3 * c3 ** 2 + b4 * c4 ** 2 - sp.Rational(1,3)
eq4 = b2 * c2 ** 3 + b3 * c3 ** 3 + b4 * c4 ** 3 - sp.Rational(1,4)
eq5 = b3 * a32 * c2 + b4 * a42 * c2 + b4 * a43 * c3 - sp.Rational(1,6)
eq6 = b3 * a32 * c3 * c2 + b4 * c4 * a42 * c2 + b4 * c4 * a43 * c3 - sp.Rational(1,8)
eq7 = b3 * a32 * c2 ** 2 + b4 * a42 * c2 ** 2 + b4 * a43 * c3 ** 2 - sp.Rational(1,12)
eq8 = c2 - a21
eq9 = c3 - a31 - a32
eq10 = c4 - a41 - a42 - a43
# Solve the order conditions
sp.solve((eq1, eq2, eq3, eq4, eq5, eq6, eq7, eq8, eq9, eq10))
% Declare symbolic variables
syms a21 a31 a32 a41 a42 a43 b1 b2 b3 b4
% Define c values
c2 = 1/4;
c3 = 1/2;
c4 = 1;
% Define order conditions
eq1 = b1 + b2 + b3 + b4 == 1;
eq2 = b2 * c2 + b3 * c3 + b4 * c4 == 1/2;
eq3 = b2 * c2^2 + b3 * c3^2 + b4 * c4^2 == 1/3;
eq4 = b2 * c2^3 + b3 * c3^3 + b4 * c4^3 == 1/4;
eq5 = b3 * a32 * c2 + b4 * a42 * c2 + b4 * a43 * c3 == 1/6;
eq6 = b3 * a32 * c3 * c2 + b4 * c4 * a42 * c2 + b4 * c4 * a43 * c3 == 1/8;
eq7 = b3 * a32 * c2^2 + b4 * a42 * c2^2 + b4 * a43 * c3^2 == 1/12;
eq8 = c2 - a21;
eq9 = c3 - a31 - a32;
eq10 = c4 - a41 - a42 - a43;
% Solve order conditions
solve(eq1, eq2, eq3, eq4, eq5, eq6, eq7, eq8, eq9, eq10)
Output:
{a31: 0,
a32: 1/2,
a41: 1,
a42: -2,
a43: 2,
b1: 1/6,
b2: 0,
b3: 2/3,
b4: 1/6,
a21: 1/4}
The Butcher tableau for this method is
Solution to Exercise 2.7
The \(t_n\) values are
Using the second-order ERK method derived in Exercise 2.3
So the solution is
Solution to Exercise 2.8
Solution
| t | y | Exact |
|:----:|:---------:|:---------:|
| 0.00 | 1.000000 | 1.000000 |
| 0.40 | 0.760000 | 0.740640 |
| 0.80 | 0.724800 | 0.698658 |
| 1.20 | 0.828864 | 0.802388 |
| 1.60 | 1.027628 | 1.003793 |
| 2.00 | 1.290787 | 1.270671 |
Plot
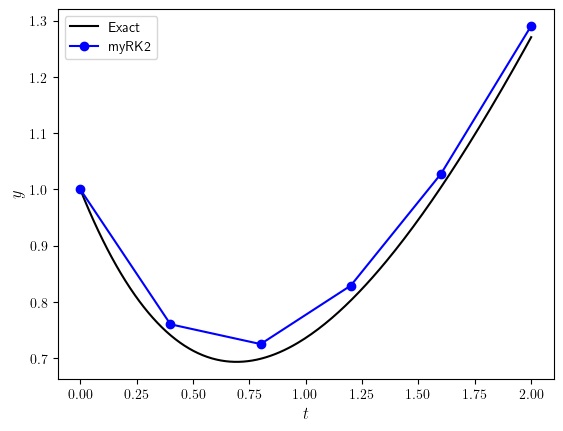
Code
import numpy as np
import matplotlib.pyplot as plt
def myrk2(f, t, y, h):
k1 = f(t, y)
k2 = f(t + 3/4 * h, y + 3 / 4 * h * k1)
return y + h / 3 * (k1 + 2 * k2)
def solveIVP(f, tspan, y0, h, solver):
# Initialise t and y arrays
t = np.arange(tspan[0], tspan[1] + h, h)
y = np.zeros((len(t),len(y0)))
t[0] = tspan[0]
y[0,:] = y0
# Loop through steps and calculate single step solver solution
for n in range(len(t) - 1):
y[n+1,:] = solver(f, t[n], y[n,:], h)
return t, y
# Define ODE function and exact solution
def f(t, y):
return t - y
def exact(t):
return t + 2 * np.exp(-t) - 1
# Define IVP
tspan = [0, 2] # boundaries of the t domain
y0 = [1] # solution at the lower boundary
h = 0.4 # step length
# Calculate the solution to the IVP
t, y = solveIVP(f, tspan, y0, h, myrk2)
# Print table of solution values
print("| t | y | Exact |")
print("|:----:|:---------:|:---------:|")
for n in range(len(t)):
print(f"| {t[n]:4.2f} | {y[n,0]:9.6f} | {exact(t[n]):9.6f} |")
# Plot solutions
t_exact = np.linspace(tspan[0], tspan[1], 200)
y_exact = exact(t_exact)
fig, ax = plt.subplots()
plt.plot(t_exact, y_exact, "k", label="Exact")
plt.plot(t, y, "bo-", label="myRK2")
plt.xlabel("$t$", fontsize=12)
plt.ylabel("$y$", fontsize=12)
plt.legend()
plt.show()
% Define ODE function and exact solution
f = @(t, y) t - y;
exact = @(t) t + 2 * exp(-t) - 1;
% Define IVP parameters
tspan = [0, 2]; % boundaries of the t domain
y0 = 1; % initial value of the solution
h = 0.4; % step length
% Calculate the solution to the IVP
[t, y_myrk2] = solveIVP(f, tspan, y0, h, @myrk2);
% Calculate exact solution for plotting
texact = linspace(tspan(1), tspan(2), 200);
yexact = exact(texact);
% Print table of solution values (for loop is used to group print statements)
for i = 1 : 1
fprintf('| t | y | Exact |\n|:----:|:---------:|:---------:|');
for n = 1 : length(t)
fprintf('\n| %4.2f | %9.6f | %9.6f |', t(n), y_myrk2(n), exact(t(n)));
end
end
% Plot solution
plot(texact, yexact, 'k-', LineWidth=2)
hold on
plot(t, y_myrk2, 'b-o', LineWidth=2, MarkerFaceColor='b')
hold off
axis padded
xlabel('$t$', FontSize=14, Interpreter='latex')
ylabel('$y$', FontSize=14, Interpreter='latex')
legend('Exact', 'myRK2', Location='northwest', FontSize=12)
% ----------------------------------------------------------------------------
function [t, y] = solveIVP(f, tspan, y0, h, solver)
% Define t and y arrays
t = (tspan(1) : h : tspan(2));
y = zeros(length(t), length(y0));
y(1,:) = y0;
% Loop through the steps and calculate single step solver solution
for n = 1 : length(t) - 1
y(n+1,:) = solver(f, t(n), y(n,:), h);
end
end
% ----------------------------------------------------------------------------
function ynew = myrk2(f, t, y, h)
k1 = f(t, y);
k2 = f(t + 3/4 * h, y + 3/4 * h * k1);
ynew = y + h / 3 * (k1 + 2 * k2);
end
Solution to Exercise 2.9
Plot
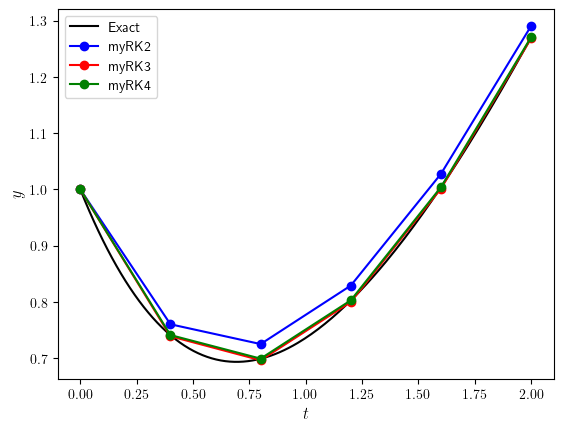
Code
import numpy as np
import matplotlib.pyplot as plt
def myrk2(f, t, y, h):
k1 = f(t, y)
k2 = f(t + 3/4 * h, y + 3 / 4 * h * k1)
return y + h / 3 * (k1 + 2 * k2)
def myrk3(f, t, y, h):
k1 = f(t, y)
k2 = f(t + 1/2 * h, y + 1/2 * h * k1)
k3 = f(t + h, y + h * (-k1 + 2 * k2))
return y + h / 6 * (k1 + 4 * k2 + k3)
def myrk4(f, t, y, h):
k1 = f(t, y)
k2 = f(t + 1/4 * h, y + 1/4 * h * k1)
k3 = f(t + 1/2 * h, y + 1/2 * h * k2)
k4 = f(t + h, y + h * (k1 - 2 * k2 + 2 * k3))
return y + h / 6 * (k1 + 4 * k3 + k4)
def solveIVP(f, tspan, y0, h, solver):
# Initialise t and y arrays
t = np.arange(tspan[0], tspan[1] + h, h)
y = np.zeros((len(t),len(y0)))
t[0] = tspan[0]
y[0,:] = y0
# Loop through steps and calculate single step solver solution
for n in range(len(t) - 1):
y[n+1,:] = solver(f, t[n], y[n,:], h)
return t, y
# Define ODE function and exact solution
def f(t, y):
return t - y
def exact(t):
return t + 2 * np.exp(-t) - 1
# Define IVP
tspan = [0, 2] # boundaries of the t domain
y0 = [1] # solution at the lower boundary
h = 0.4 # step length
# Calculate the solution to the IVP
t, y_myrk2 = solveIVP(f, tspan, y0, h, myrk2)
t, y_myrk3 = solveIVP(f, tspan, y0, h, myrk3)
t, y_myrk4 = solveIVP(f, tspan, y0, h, myrk4)
# Plot solutions
t_exact = np.linspace(tspan[0], tspan[1], 200)
y_exact = exact(t_exact)
fig, ax = plt.subplots()
plt.plot(t_exact, y_exact, "k", label="Exact")
plt.plot(t, y_myrk2, "bo-", label="myRK2")
plt.plot(t, y_myrk3, "ro-", label="myRK3")
plt.plot(t, y_myrk4, "go-", label="myRK4")
plt.xlabel("$t$", fontsize=12)
plt.ylabel("$y$", fontsize=12)
plt.legend()
plt.show()
% Define ODE function and exact solution
f = @(t, y) t - y;
exact = @(t) t + 2 * exp(-t) - 1;
% Define IVP parameters
tspan = [0, 2]; % boundaries of the t domain
y0 = 1; % initial value of the solution
h = 0.4; % step length
% Calculate the solution to the IVP
[t, y_myrk2] = solveIVP(f, tspan, y0, h, @myrk2);
[t, y_myrk3] = solveIVP(f, tspan, y0, h, @myrk3);
[t, y_myrk4] = solveIVP(f, tspan, y0, h, @myrk4);
% Calculate exact solution for plotting
texact = linspace(tspan(1), tspan(2), 200);
yexact = exact(texact);
% Plot solution
plot(texact, yexact, 'k-', LineWidth=1)
hold on
plot(t, y_myrk2, 'b-o', LineWidth=2, MarkerFaceColor='b')
plot(t, y_myrk3, 'r-o', LineWidth=2, MarkerFaceColor='r')
plot(t, y_myrk4, 'g-o', LineWidth=2, MarkerFaceColor='g')
hold off
axis padded
xlabel('$t$', FontSize=14, Interpreter='latex')
ylabel('$y$', FontSize=14, Interpreter='latex')
legend('Exact', 'myRK2', 'myRK3', 'myRK4', Location='northwest', FontSize=12)
% ----------------------------------------------------------------------------
function [t, y] = solveIVP(f, tspan, y0, h, solver)
% Define t and y arrays
t = (tspan(1) : h : tspan(2));
y = zeros(length(t), length(y0));
y(1,:) = y0;
% Loop through the steps and calculate single step solver solution
for n = 1 : length(t) - 1
y(n+1,:) = solver(f, t(n), y(n,:), h);
end
end
% ----------------------------------------------------------------------------
function ynew = myrk2(f, t, y, h)
k1 = f(t, y);
k2 = f(t + 3/4 * h, y + 3/4 * h * k1);
ynew = y + h / 3 * (k1 + 2 * k2);
end
% ----------------------------------------------------------------------------
function ynew = myrk3(f, t, y, h)
k1 = f(t, y);
k2 = f(t + 1/2 * h, y + 1/2 * h * k1);
k3 = f(t + h, y + h * (-k1 + 2 * k2));
ynew = y + h / 6 * (k1 + 4 * k2 + k3);
end
% ----------------------------------------------------------------------------
function ynew = myrk4(f, t, y, h)
k1 = f(t, y);
k2 = f(t + 1/4 * h, y + 1/4 * h * k1);
k3 = f(t + 1/2 * h, y + 1/2 * h * k2);
k4 = f(t + h, y + h * (k1 - 2 * k2 + 2 * k3));
ynew = y + h / 6 * (k1 + 4 * k3 + k4);
end
Solution to Exercise 2.10
Global truncation errors
| h | myRK2 | myRK3 | myRK4 |
|:-----:|:--------:|:--------:|:--------:|
| 0.400 | 2.01e-02 | 1.99e-03 | 1.61e-04 |
| 0.200 | 4.23e-03 | 2.12e-04 | 8.53e-06 |
| 0.100 | 9.74e-04 | 2.44e-05 | 4.90e-07 |
| 0.050 | 2.34e-04 | 2.93e-06 | 2.94e-08 |
| 0.025 | 5.75e-05 | 3.60e-07 | 1.80e-09 |
Plot
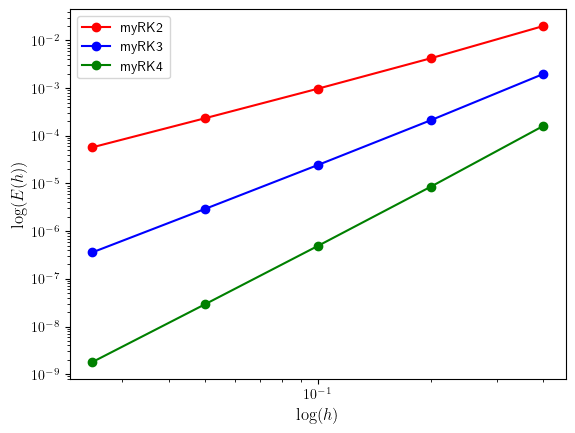
Estimating the order of the methods
Code
# Calculate solution for decreasing step lengths
hvals = [0.4, 0.2, 0.1, 0.05, 0.025]
tval = 2
E_myrk2, E_myrk3, E_myrk4 = [], [], []
for h in hvals:
t, y_myrk2 = solveIVP(f, tspan, y0, h, myrk2)
t, y_myrk3 = solveIVP(f, tspan, y0, h, myrk3)
t, y_myrk4 = solveIVP(f, tspan, y0, h, myrk4)
idx = np.argmin(abs(t - tval))
E_myrk2.append(abs(exact(tval) - y_myrk2[idx,0]))
E_myrk3.append(abs(exact(tval) - y_myrk3[idx,0]))
E_myrk4.append(abs(exact(tval) - y_myrk4[idx,0]))
# Output table of errors
print("| h | myRK2 | myRK3 | myRK4 |")
print("|:-----:|:--------:|:--------:|:--------:|")
for n in range(len(hvals)):
print(f"| {hvals[n]:0.3f} | {E_myrk2[n]:0.2e} | {E_myrk3[n]:0.2e} | {E_myrk4[n]:0.2e} |")
# Plot errors on a log scale
fig, ax = plt.subplots()
plt.loglog(hvals, E_myrk2, 'ro-', label="myRK2")
plt.loglog(hvals, E_myrk3, 'bo-', label="myRK3")
plt.loglog(hvals, E_myrk4, 'go-', label="myRK4")
plt.xlabel(r"$\log(h)$", fontsize=12)
plt.ylabel(r"$\log(E(h))$", fontsize=12)
plt.legend()
plt.show()
% Calculate solution for decreasing step lengths
hvals = [0.4, 0.2, 0.1, 0.05, 0.025];
tval = 2;
E_myrk2 = [];
E_myrk3 = [];
E_myrk4 = [];
for h = hvals
[t, y_myrk2] = solveIVP(f, tspan, y0, h, @myrk2);
[t, y_myrk3] = solveIVP(f, tspan, y0, h, @myrk3);
[t, y_myrk4] = solveIVP(f, tspan, y0, h, @myrk4);
[~, idx] = min(abs(tval - t));
E_myrk2 = [E_myrk2, abs(exact(tval) - y_myrk2(idx))];
E_myrk3 = [E_myrk3, abs(exact(tval) - y_myrk3(idx))];
E_myrk4 = [E_myrk4, abs(exact(tval) - y_myrk4(idx))];
end
% Output table of errors (for loop is used to group print statements)
for i = 1 : 1
fprintf('| t | myRK2 | myRK4 | myRK4 |')
fprintf('|:-----:|:--------:|:--------:|:--------:|');
for n = 1 : length(hvals)
fprintf('\n| %1.3f | %1.2e | %1.2e | %1.2e |', hvals(n), E_myrk2(n), E_myrk3(n), E_myrk4(n))
end
end
% Plot errors on a loglog scale
loglog(hvals, E_myrk2, 'ro-', MarkerFaceColor='r', LineWidth=2)
hold on
loglog(hvals, E_myrk3, 'bo-', MarkerFaceColor='b', LineWidth=2)
loglog(hvals, E_myrk4, 'go-', MarkerFaceColor='g', LineWidth=2)
hold off
axis padded
xlabel('$\log(h)$', FontSize=14, Interpreter='latex')
ylabel('$\log(E(h))$', FontSize=14, Interpreter='latex')
legend('myRK2', 'myRK3', 'myRK4', Location='southeast', FontSize=12)
Solution to Exercise 2.11
Plot
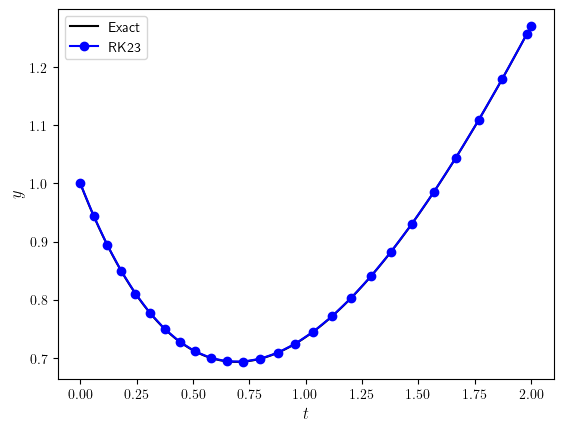
There were 26 successful steps and 3 failed steps.
Code
import numpy as np
import matplotlib.pyplot as plt
def solveIVP_SSC(f, tspan, y0, h, solver, tol=1e-4):
# Define t and y arrays
t = np.zeros(10000)
y = np.zeros((10000,len(y0)))
t[0] = tspan[0]
y[0,:] = y0
# Solver loop
n = 0
while t[n] < tspan[-1]:
# Ensure t does not exceed tmax
h = min(h, tspan[-1] - t[n])
# Calculate order p+1 and p solutions
yp1, yp, order = solver(f, t[n], y[n,:], h)
# Determine whether step was successful or not
delta = np.max(np.abs(yp1 - yp))
if delta < tol:
y[n+1,:] = yp1
t[n+1] = t[n] + h
n += 1
# Calculate new value of h
h *= max(0.5, min(2, 0.9 * (tol / delta) ** (1 / (order + 1))))
return t[:n+1], y[:n+1,:]
def rk23(f, t, y, h):
k1 = f(t, y)
k2 = f(t + 1/2 * h, y + 1/2 * h * k1)
k3 = f(t + h, y + h * (-k1 + 2 * k2))
y3 = y + h / 6 * (k1 + 4 * k2 + k3)
y2 = y + h * k2
return y3, y2, 2
# Define ODE function and exact solution
def f(t, y):
return t - y
def exact(t):
return t + 2 * np.exp(-t) - 1
# Define IVP
tspan = [0, 2] # boundaries of the t domain
y0 = [1] # solution at the lower boundary
h = 0.4 # step length
tol = 1e-4 # accuracy tolerance
# Calculate the solution to the IVP
t, y = solveIVP_SSC(f, tspan, y0, h, rk23, tol)
# Plot solutions
t_exact = np.linspace(tspan[0], tspan[1], 200)
y_exact = exact(t_exact)
fig, ax = plt.subplots()
plt.plot(t_exact, y_exact, "k", label="Exact")
plt.plot(t, y, "bo-", label="RK23")
plt.xlabel("$t$", fontsize=12)
plt.ylabel("$y$", fontsize=12)
plt.legend()
plt.show()
% Define ODE function and exact solution
f = @(t, y) t - y;
exact = @(t) t + 2 * exp(-t) - 1;
% Define IVP parameters
tspan = [0, 2]; % boundaries of the t domain
y0 = 1; % initial value of the solution
h0 = 0.4; % step length
tol = 1e-4; % accuracy tolerance
% Calculate the solution to the IVP
[t, y] = solveIVP_SSC(f, tspan, y0, h0, @rk23, tol);
% Output number of successful steps
fprintf("%0i successful steps", length(t) - 1)
% Calculate exact solution for plotting
texact = linspace(tspan(1), tspan(2), 200);
yexact = exact(texact);
% Plot solution
plot(texact, yexact, 'k-', LineWidth=2)
hold on
plot(t, y, 'b-o', LineWidth=2, MarkerFaceColor='b')
hold off
axis padded
xlabel('$t$', FontSize=14, Interpreter='latex')
ylabel('$y$', FontSize=14, Interpreter='latex')
legend('Exact', 'myRK2', Location='northwest', FontSize=12)
% ----------------------------------------------------------------------------
function [t, y] = solveIVP_SSC(f, tspan, y0, h, solver, tol)
% Define t and y arrays
t = zeros(10000);
y = zeros(10000, length(y0));
t(1) = tspan(1);
y(1,:) = y0;
% Loop through the steps
n = 1;
while t(n) < tspan(2)
% Ensure t does not exceed tmax
h = min(h, tspan(2) - t(n));
% Calculate order p and p+1 solutions
[yp1, yp, p] = solver(f, t(n), y(n,:), h);
% Determine whether the step was successful or not
delta = max(abs(yp1 - yp));
if delta < tol
y(n+1,:) = yp1;
t(n+1) = t(n) + h;
n = n + 1;
end
% Calculate new value of h
h = h * max(0.5, min(2, 0.9 * (tol / delta) ^ (1 / (p + 1))));
end
% Remove unused entries from t and y
t(n+1:end) = [];
y(n+1:end,:) = [];
% Print number of successful and failed steps
fprintf("%1i successful steps\n%1i failed steps", nsucc, nfail)
end
% ----------------------------------------------------------------------------
function [y3, y2, order] = rk23(f, t, y, h)
k1 = f(t, y);
k2 = f(t + 1/2 * h, y + 1/2 * h * k1);
k3 = f(t + h, y + h * (-k1 + 2 * k2));
y3 = y + h * (1/6 * k1 + 2/3 * k2 + 1/6 * k3);
y2 = y + h * k2;
order = 2
end